Ensuring accuracy and validity
We know the importance of data quality when drawing conclusions about an organisation’s ESG performance and sustainability initiatives (check out our previous blog post about data accuracy and timeliness). When AI is used to generate these insights, it’s even more important. Inaccurate, out-of-date, or incomplete data can lead to biased outcomes, potentially generating misleading assessments. Emphasising data quality allows businesses to reduce the risk of misinterpretation, false positives and distorted conclusions. This is essential in building trust in AI-based sustainability analysis!
Mitigating bias and the ethical concerns of AI
As we just mentioned, one of the main concerns regarding the use of AI in business is the possibility of increased bias. Unrepresentative or subjective data can perpetuate existing inequalities or reinforce systemic biases. This can then go against the good work of the social sustainability initiatives set out by your business. Implementing rigorous data collection methods and ensuring diversity and inclusivity in the data can help minimise this bias and enhance the fairness of AI-driven ESG assessments.
Holding ourselves accountable
Transparency and accountability are often overlooked as key elements of quality data. It’s important that businesses can provide evidence of data provenance and audit trails of collection. For example, a thorough and accurate carbon footprint methodology. Data collection processes should be documented transparently and be accessible to relevant stakeholders. Without this, it can be unclear why decisions were made. Keeping accurate methodologies can help build a culture of accountability by promoting an understanding of how and why assessments are made.
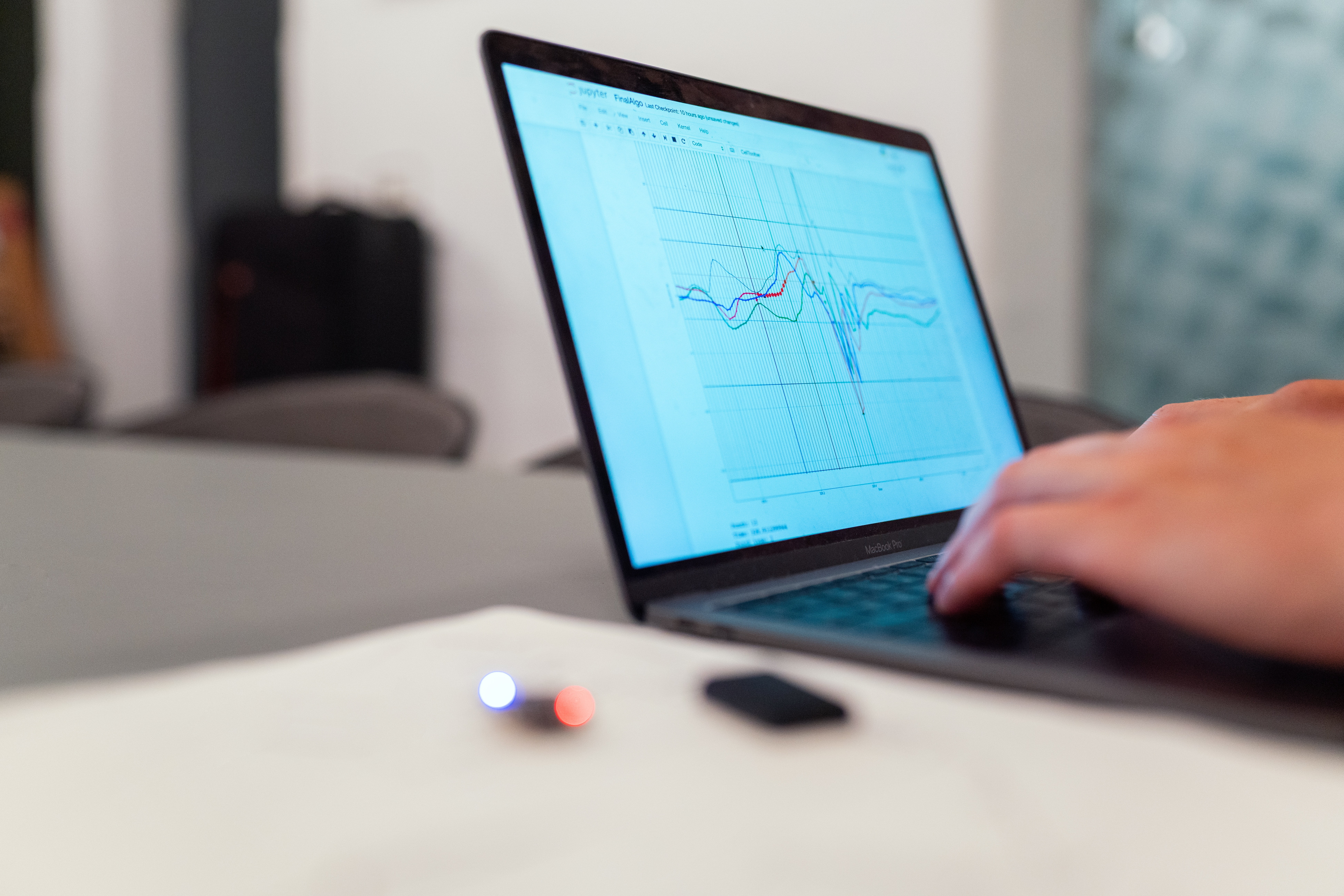
What are the potential hurdles that organisations encounter in ensuring data quality?
Data quality is often something that is ‘easier said than done’. The challenges of ensuring high-quality data for AI models are like those you may have faced when collecting non-financial data previously. Collecting and cleaning information from different sources can be difficult and time-consuming; especially when attempting to eradicate duplicates, handle non-numerical ‘categorical’ variables and meet a universal standard when there are so many potential sources of error to consider. Implementing ML-based predictive analytics also faces the challenges of requiring extensive datasets, often multiple datasets with different structures. A common example is different data sets encompassing each scope 1, 2, and 3 carbon emissions. Collating these within an ensemble can lead to very complex systems, which can be a big challenge to evaluate and score their accuracy.
A source of detriment to data quality that we see often is data estimation. If a data point has been estimated, and it has not been tagged as estimated, or the estimation method is not clear, the reliability of that data point becomes very questionable. This plays back into the idea of ‘garbage in, garbage out’: a numerical ML model trained on estimated data will create an output probably no more credible than guesswork.
As we’ve previously mentioned, it’s also key to ensure your initial data set is representative and objective. This may mean you need to evaluate your data collection process. Organisations can also find it difficult to implement proper data governance systems and processes. Not only does this tie into the importance of accountability and transparency, but poor data management can also lead to inconsistencies, errors, and bias in the data.
Addressing these issues often requires external support…
This may be in the form of a data quality tool or team to monitor and check data quality. Having dedicated resource that can clean, validate and check data can make a huge difference to the quality of your data and thus the readiness of your organisation for the utilisation of AI. We’ve found that teams may need that extra bit of help with managing their data and ensuring it’s of high quality. If you’re interested in using AI tools for your sustainability assessments, reviewing the quality of your data should be the first thing you do! With our extensive data services, our team of experts can help you whatever the nature of your non-financial data.