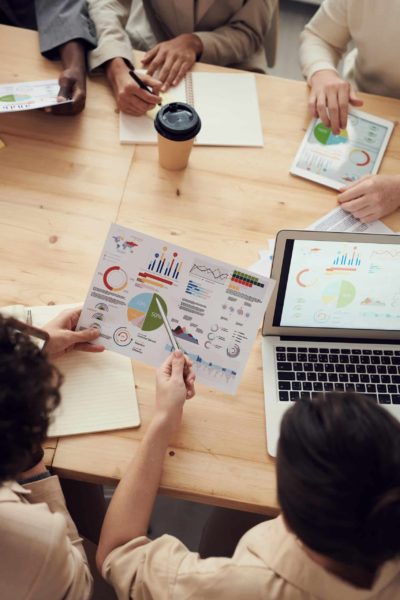
The importance of data accuracy and timeliness
The two fundamental elements of sustainable and non-financial data quality assessment: timeliness and accuracy.
Big data and sustainability
Before we dive into the importance of data accuracy, we just want to outline the wider role of Big data in sustainability. Big data drives so much of the world we live in that it’s almost hard to perceive of a world before it arrived. But to think of big data as a new phenomenon is misleading. The data has always been there, ready and waiting, for us to harvest. What we’ve lacked until now are the tools, and to some degree the motivation, to harvest it on a truly massive scale.
Nowadays big data is playing a significant role in sustainability practices in the manufacturing industries, but also across many other sectors. To some extent this has been driven by compliance and regulation, but there is no doubt that the drive to develop a sophisticated and far reaching corporate social responsibility programme is driving profitability and competitiveness in the global marketplace. As the tools we have access to develop, so too does the quality of data we have the potential to harvest with them.
To a large degree the evolution of sensors, beacons, GPS and other data recording technology sat within the Internet of Things, has driven our ability to capture large swaths of data. But ascertaining the importance and even relevance of all this data means understanding firstly its quality. Because, just like any other business asset, data should be treated as such and accorded the same kind of quality control.
But big data is nothing if it is of poor quality. Like any mined resource, data needs to be of high quality to deliver on the initial investment in getting it out the ground (or in our case the supply chain). Let’s explore what I mean by data quality.
The dimensions of data quality
Collecting and then managing huge amounts of data is an enormous challenge for any organisation. Key to managing data is in qualifying and guaranteeing the quality of that data so it can be interpreted and acted upon. For data to be high quality it must be, according to the academic Tom Redman, defined as “fit for their intended uses in operations, decision making and planning”.
Two crucial dimensions of data quality are timeliness and accuracy (which we’ll look at in a minute) but all data can be defined as having six quality dimensions. Each of these can be understood through certain questions relating to the data:
- Completeness: Is all the intended data being produced in the data set or is any of it missing?
- Uniqueness: Are any individual pieces of data from the dataset recorded more than once?
- Timeliness: How long is the time difference between data capture and the real world event being captured?
- Validity: Is the data presented in the correct and pre-defined format, type or range so as to be applicable to the given analytical task?
- Accuracy: Does the data matches up with the real world object or event it describes, enabling correct conclusions to be drawn from it?
- Consistency: Is the given dataset consistent and correlative with different representations of the same information across multiple datasets?

Why data accuracy and timeliness matter
In the field of sustainability data management, timeliness and accuracy are fundamental prerequisites when it comes to data analysis. Failings in either of these dimensions can compromise the usefulness of your data. Let’s look at each in turn.
Data timeliness
Timing is everything and when you’re capturing, interpreting and then acting on real-time data timeliness can be fundamental. Let’s imagine a scenario in which we’re measuring energy input and some form of output from a manufacturing process. If we were to precisely record our energy input against our output then we could conceivably find the optimum collaboration and adjust accordingly.
Now imagine this system has several inputs and several outputs. Suddenly the importance of data timeliness comes into play. In a dynamic and rapidly evolving system, a second or even a few microseconds between one reading and another can mean one dataset is mismatched against another. In this example it’s essential to ensure that all our data is timely and being interpreted in real-time to ensure the best optimisation of the system.
Data accuracy
The second pillar of strong and reliable sustainability data is accuracy. This is the degree to which the data reflects the real world. Ensuring data accuracy isn’t always easy as slight inaccuracies will not paint an entirely unfeasible picture and therefore comparison between ‘real life’ and the dataset will not give cause for concern. In many data driven processes though, a high degree of accuracy can make all the difference, especially over long periods of time, when small inaccuracies can equate to quite fundamental inefficiencies.
Ensuring data accuracy isn’t always easy. Proper configuration of sensor or recording equipment is vital but a reference point is also needed in many cases. This will usually take form of a separate third party dataset from the same time period that can be relied on. Close inspection of how a given dataset relates to and fits with other related datasets can also help to identify inaccuracies. In other words if one dataset produces totally unexpected results based on the findings of another dataset then it’s likely one of those datasets is inaccurate. Establishing a robust data validation process with data accuracy rules and acceptable margins of error is therefore essential.
How sustainability software can help with data accuracy and timeliness
Using sustainability data management software is one way of bringing disparate datasets together but it is also a powerful tool for establishing timeliness and accuracy. With data validation such a crucial facet of the data management process, migrating and unifying date under one system can drastically save time and effort in comparing datasets for timeliness and accuracy issues and in some cases even automate the process entirely. This can require resource, time or expertise that your team may need. We can help you with data management, verification and more.