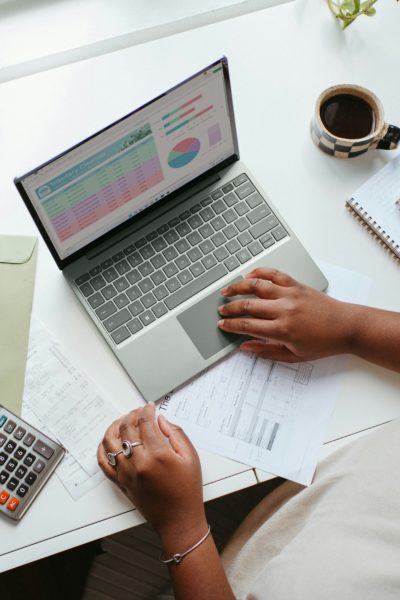
Latest news & insights
March 31st 2025 by Pip Stockford
Why your SME should invest in sustainability data in 2025
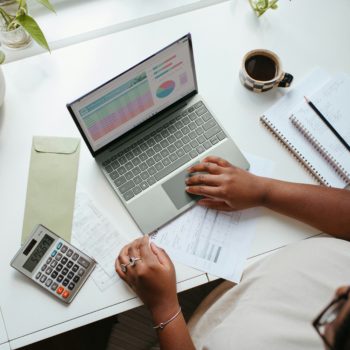
Why your SME should invest in sustainability data in 2025
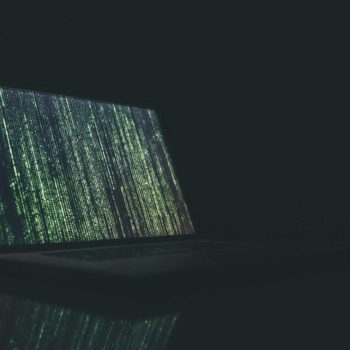
The Intersection of Cybersecurity and ESG: Building Resilience for Sustainable Success
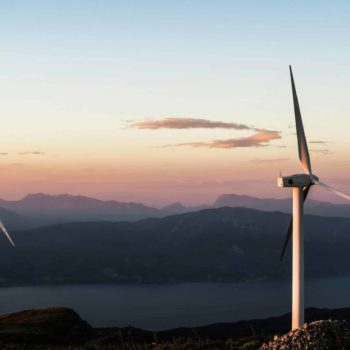
ISO Net Zero Standard: What you need to know
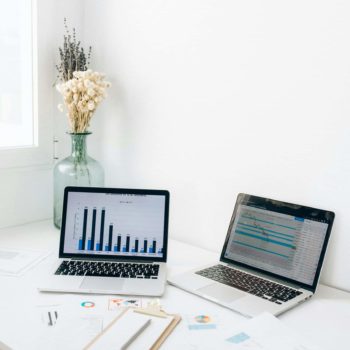
Our 5 Simple Steps to CSRD Reporting
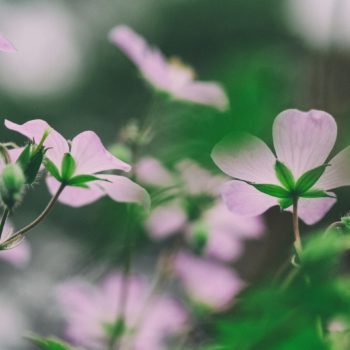
ESG Trends for 2025
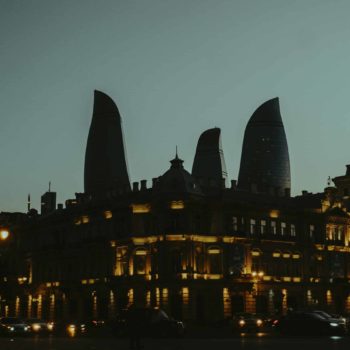
COP29 debrief – Tensions and temperatures rise
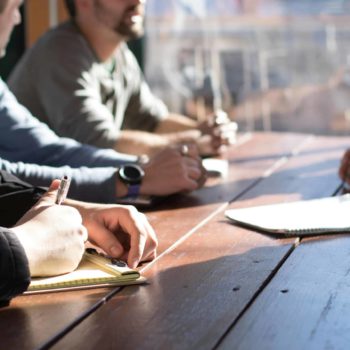
CSRD: How to Engage with Stakeholders
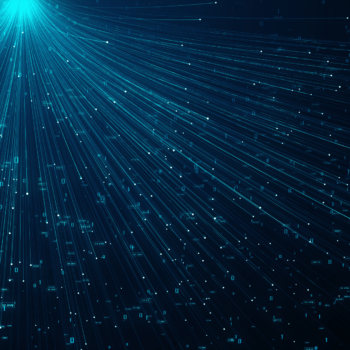
AI and Data for Effective ESG Reporting: Insights from Risk London
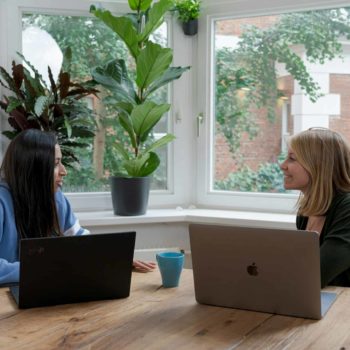
Making the Business Case for Sustainability

Insights from the ESS Expo 2024
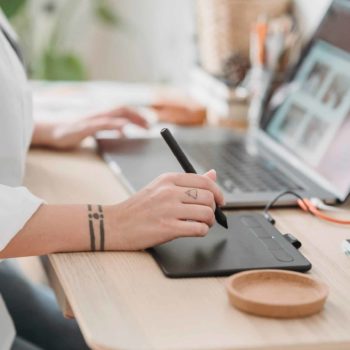
The power of data visualisation
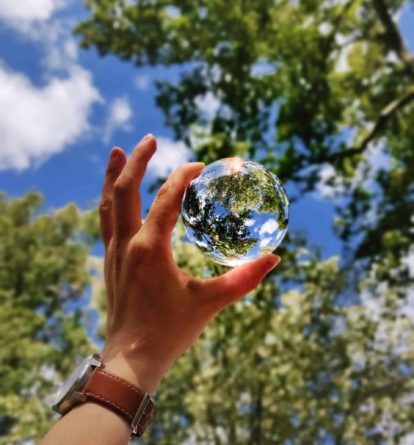
Want to hear more?