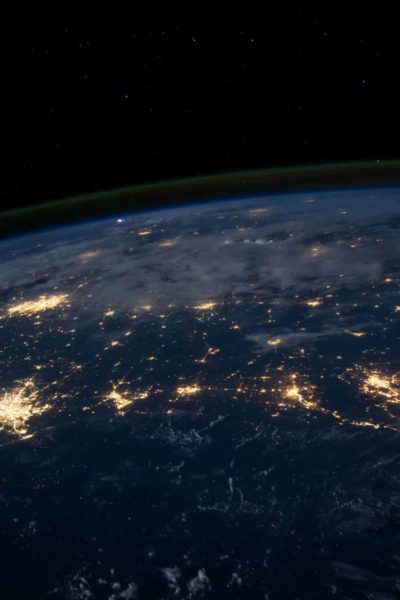
The 6 dimensions of data quality – the key to sustainable success
The volume of data being collected across the world continues to grow exponentially, thanks to technological advancements and our ability to gather real-time information. Yet having all this data doesn’t mean much if it’s of poor quality. With such an overwhelming amount of information at our fingertips, it’s crucial to ensure that the data is meaningful and can be used to support any decisions, comparisons and statements…
Consumers are becoming increasingly conscious of the impact of the products and services they’re using. Simultaneously, reporting requirements and legislation are growing increasingly stringent. High-quality data can help you stay ahead of regulations, support your green claims and evidence your commitment to sustainability.
But what does data quality mean? It’s important to get back to basics to understand whether your sustainability data is high quality or not. In this blog post, we’re going to summarise the 6 dimensions of data quality, and how they can apply to business sustainability.
1. Completeness: Is all the intended data being collected or are there areas missing?
Completeness refers to how well the data covers a time period, and the breadth of the information captured. For lots of sustainability initiatives, it is important to be able to see the impact of a particular measure across the whole of an organisation. Completeness of data is crucial in this regard as it determines whether the data covers all aspects of the business. This might include offices, factories, and suppliers. You also want to consider whether the data has been collected over multiple years/months, in order to provide confidence in the data and allow comparisons over time. Ensure that you collect enough data to provide evidence for decisions, especially when setting sustainability goals.
2. Uniqueness: Are any individual pieces of data from the dataset recorded more than once?
Uniqueness means that you ensure that there are no duplicates or overlaps within the data being collected. For example, let’s say there are shared waste facilities between Offices and Factories. We would need to ensure that only one of them records the amount of waste generated, so we don’t end up duplicating the data. Another example might be ensuring only one person recorded an employee accident, so there aren’t duplicate records of the same accident. Ensuring uniqueness of data points builds trust in the data and the analysis built from the data.
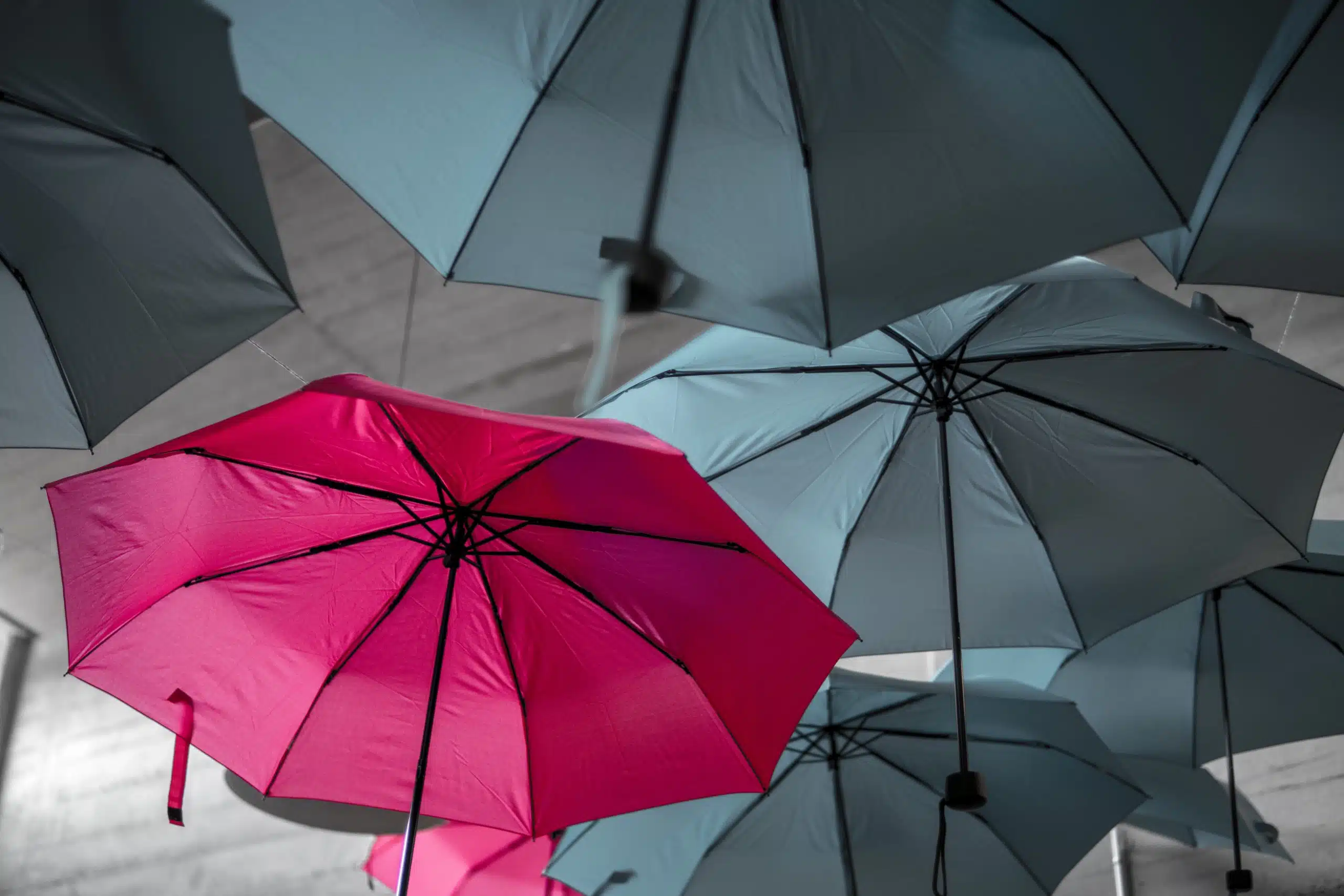
3. Validity: Is the data presented in the correct and pre-defined format, type or range so as to be applicable to the given analytical task?
For data to be valid, it must to be recorded in the correct way required for the analysis that’s taking place. For instance, is the energy consumption data each for each year all in the same unit? To make sure that your data is always top-notch, you can put validation rules in place to catch any errors before they cause problems down the line. This might include not allowing data to be submitted if it isn’t in the correct format, or creating a flag to alert when data isn’t valid.
Collecting invalid data can have serious knock-on effects. If large amounts of the data are invalid, then this can impact the completeness of the data. And, as we mentioned previously, if data is incomplete, the required analytic tasks may not be able to be carried out.
4. Timeliness: How long is the time difference between data capture and the real-world event being captured?
Why is it so integral to data quality that data collection is carried out on time and as soon as possible after the data becomes available? Data quality may diminish over time. If not collected right away people may lose the data or forget about it and end up having to provide an estimate. Estimating can lead to misleading data that won’t provide useful insights for business strategy. Timeliness can also be important for auditing purposes. Certain data covering a certain timeframe is required for audits and reporting requirements, so it is important this data is all collected on time.
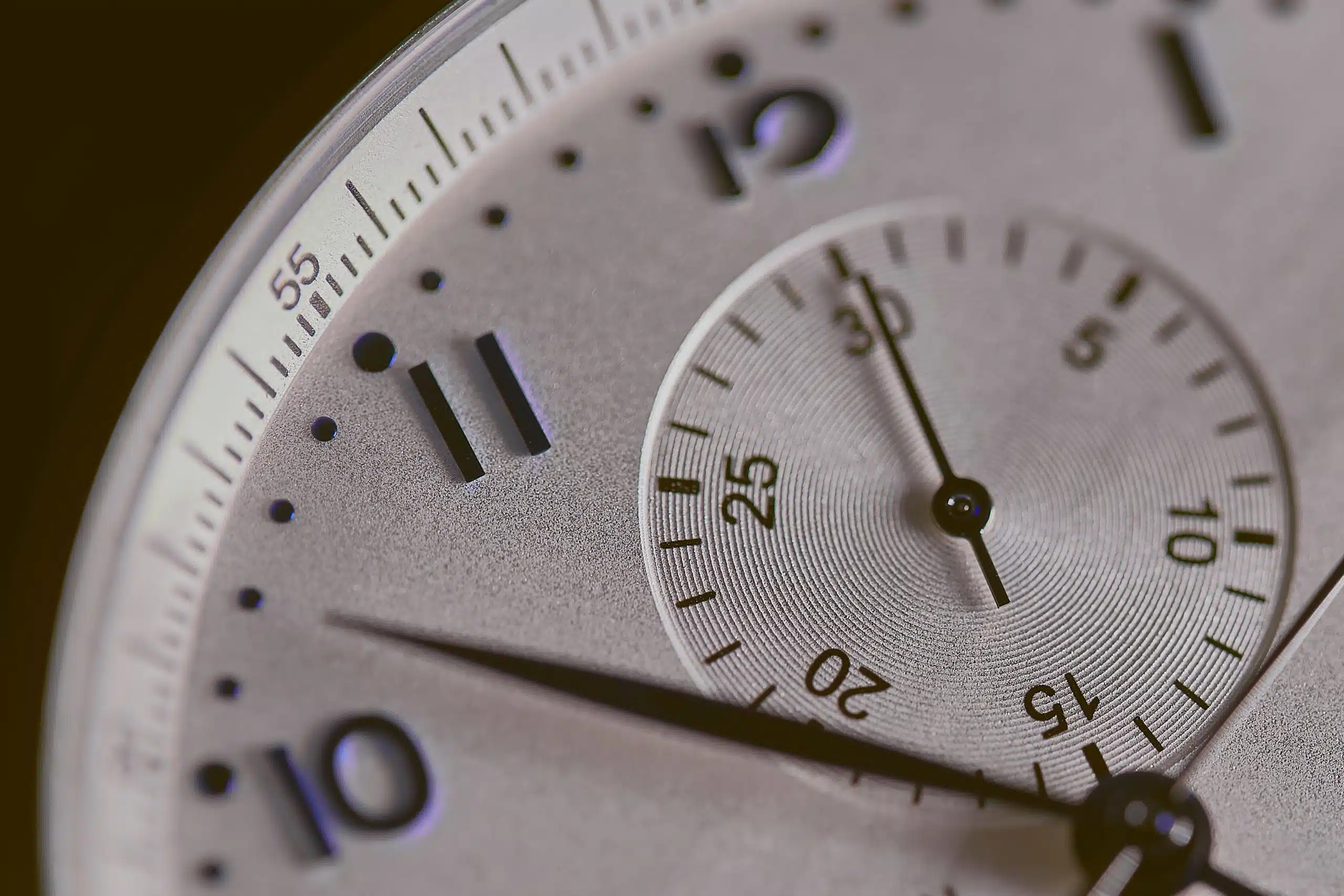
5. Accuracy: Does the data match up with the real-world object or event it describes, enabling correct conclusions to be drawn from it?
Essentially, we can think of accuracy as meaning – does that data mean what it’s supposed to in real life? It’s important that businesses find a way of ensuring that data collection can be carried out as accurately as possible. This might involve ensuring that you use the correct recording equipment and that it’s working properly. Putting validation rules and a data-checking system in place can help to identify inaccuracies. For example, if one year of data presents very different results from other years, it will be highlighted and potentially identified as an inaccurate result. In today’s world, keeping up with regulatory changes and meeting stakeholder expectations has become more important than ever. And when it comes to data, accuracy is key to building trust.
6. Consistency: Is the given dataset consistent and correlative with different representations of the same information across multiple datasets?
Put simply, consistency means that the same information stored and used at multiple instances matches up. One example of good consistency would be all sites using the same unit of measurement to track water consumption. This means that you can aggregate and analyse data from multiple sources side-by-side. It allows for comparison of data between countries and sites, and over time, to gain insights from the data. Imagine if the information was all recorded in different units, all following a slightly different data-entry policy. It would be much more difficult to understand! Consistency is the key to unlocking valuable insights from data.
In the era of information abundance, businesses need to prioritise data quality to make informed decisions, meet sustainability goals, and comply with evolving regulations…
The six dimensions of data quality – completeness, uniqueness, timeliness, validity, accuracy, and consistency – are the cornerstones of data integrity. By making sure your data adheres to these dimensions, you can harness the power of data to drive sustainability, build trust with stakeholders, and make data-driven decisions that lead to a more sustainable future. But remember, just because your data isn’t perfect, it doesn’t mean you can’t get started making a positive impact. We can help you map your data to find any gaps or issues, and then work to elevate its quality so you can extract the most valuable insights. High-quality data is the key to long-term success in a world that increasingly values transparency and environmental responsibility.
References:
https://www.gov.uk/government/news/meet-the-data-quality-dimensions
https://www.collibra.com/us/en/blog/the-6-dimensions-of-data-quality
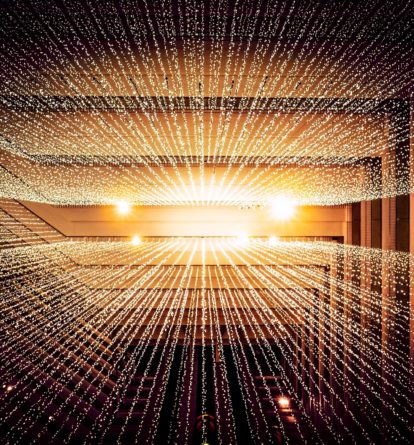